The Purge: Why Generative A.I. is Coming for SEO Content Traffic, Jobs & Dependent Websites
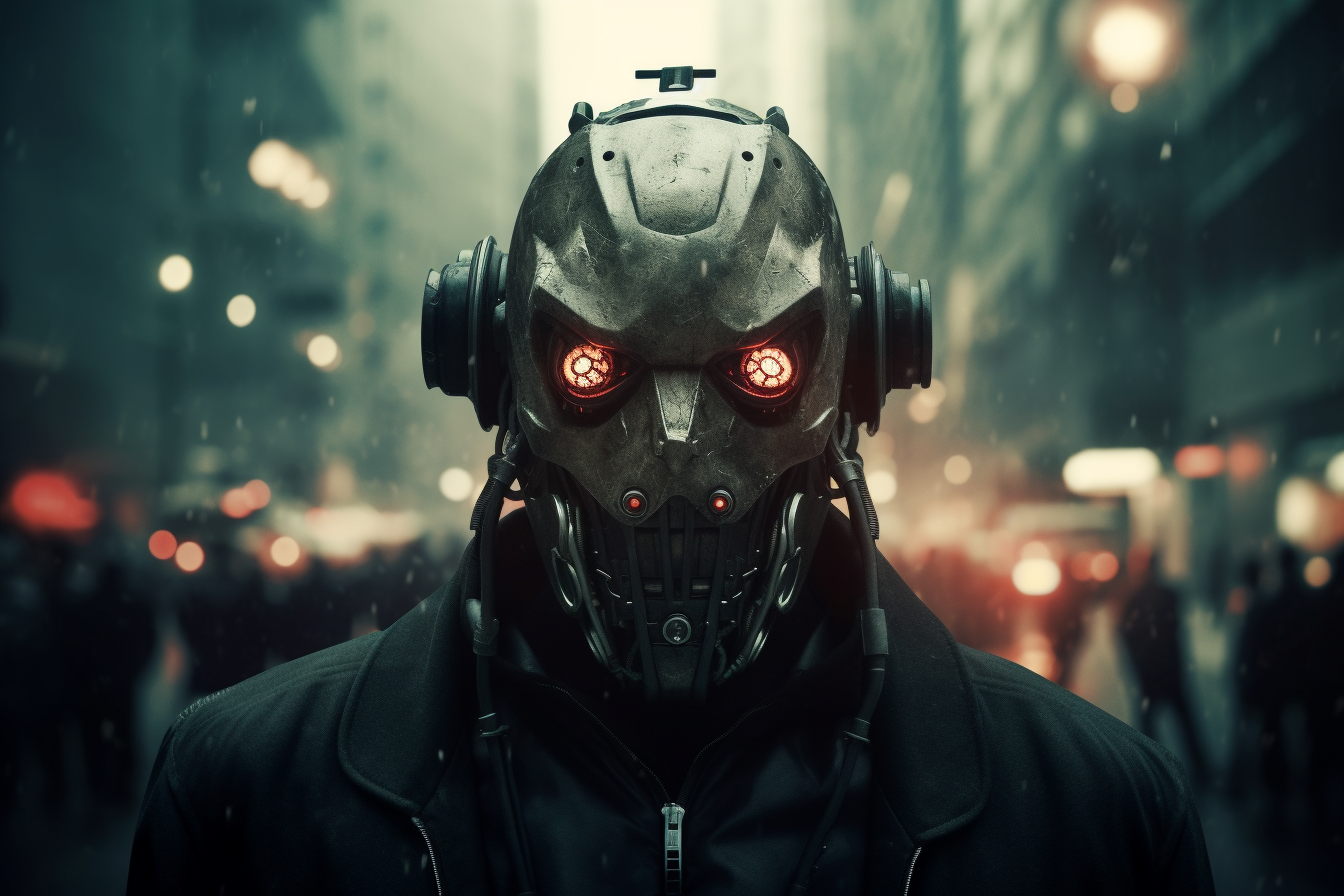
If you create content primarily for SEO purposes, or if your website is dependent on content that gets organic traffic from search engines, then there’s a very real possibility your skills and/or business will become redundant and lose significant value in the near future.
Because after what Google just announced on May 10th, showing off examples like this and this of what future SERPs might look like, Shit. Just. Got. Real.
I’m writing this so that we as an industry of SEOs and content creators collectively wake the fuck up to what’s currently unfolding, potential best/worst case scenarios of the (very near) future and what decisions we should consider making right now to adapt and survive.
Quick Disclaimers:
- I cannot predict the future.
- I myself am an SEO consultant and owner/operator of the types of sites most at risk.
- I’m U.S. based and therefore will focus most on the situation in the U.S., i.e. on regulation.
- This is a rapidly developing situation. My personal views are constantly adapting as well.
- The changes I talk about will affect some SEO content jobs/businesses more than others.
Too Long; Didn’t Read
Google, ChatGPT and others are using new generative A.I. technology to exploit your website’s content for their profit without providing any benefit to you. They’re doing so without your knowledge or consent, taking answers from your content to give to their users without them having to visit your website and without even crediting you (they don’t cite their sources). What they’re doing has currently not been declared illegal under current U.S. copyright law. And there is little to nothing you can do, as of now, to get them to stop. So as a result, unless something drastic happens, there’s a very real scenario in which you will soon see a steady, continuous decline in organic search traffic for certain types of content, and it will have nothing to do with your content’s organic search rankings.
Table of Contents
The Past: What Just Happened & How We Got Here
- What Exactly Are “Large Language Models (LLMs)”?
- Why Does Google Care About LLMs?
- What Exactly Did Google Announce on May 10th?
The Present: Important Additional Context You Should Know
- Where Do LLMs Currently Excel (and Not Excel)?
- Where do ChatGPT & Other LLMs Get Their Answers From?
- What is the Current State of U.S. Regulation on Copyright & A.I. Training Data?
- What is the Current State of the LLM Industry Landscape?
The Future: Wild Speculations About What Happens Next
- Will copyright holders get regulatory protection?
- Will LLMs continue to (rapidly) improve?
- How will the user adoption of LLMs play out from here?
- What are Google’s next moves?
- How will we as an industry respond to the threat of A.I.?
The Past: What Just Happened & How We Got Here
Let’s start by reviewing the following events:
- A new, powerful and disruptive form of A.I. was developed (“Large Language Models”).
- That technology, made famous by ChatGPT, blew up and became super popular, super quickly.
- In response, Google made some big announcements around A.I. and Search.
Let’s walk through each.
What Exactly are “Large Language Models (LLMs)”?
Any text that Google (or anyone else) labels as “Generative A.I.” is most commonly generated by a Large Language Model (LLM), a new form of artificial intelligence.
LLMs first emerged in 2018 but only became popular in the last year or so because of the emergence of the first LLM that went mainstream: OpenAI’s ChatGPT.
If you’re not familiar with ChatGPT, it’s difficult to define because its capabilities expand by the day. But for the sake of this post, we’re going to focus on the use of ChatGPT and LLMs as chatbots: you “prompt” them with a question (or command), they send back a response.
Chatbots aren’t new. But they were never really that good. At least, not until November 2022. That was when ChatGPT (Model 3.5) was released. While past versions of GPT showed promise, it wasn’t until this particular release that the potential of this new technology put the whole world on notice: ChatGPT, and Generative A.I. tools in general, are an absolute game changer.
How Do LLMs (Like ChatGPT) Work?
LLMs are complex, but I’ll try my best to explain the basic idea of how they work.
To start, an LLM begins by being given “training data”, the information it uses to “learn” from. Think: scanned pages of books, historical financial databases, copies of every Wikipedia page, etc.
It uses this corpus of information to “learn” the laws of human language and the relationships between words. We can think of this as breaking down into two key parts (although the model itself thinks of them as one combined thing, not separate):
- How humans talk/write – mainly to understand what we’re asking and how to answer it.
- Various facts, ideas, concepts, etc – the raw inputs to pull from that forms its collective “knowledge”.
The reason for #2 is obvious: if you want an answer to a question, the LLM needs to know certain facts and background information to answer it.
But #1 is the real magic of LLMs and why they’re such a technological breakthrough: by feeding it articles, forum posts, video transcriptions, etc., it can start to understand the words we use in the order we use them and what that means (as if the answer to a question is a math formula).
Once it’s done analyzing what you’re asking for, it “thinks”, and then generates a response in natural human language that (hopefully) gives you what you’re looking for.
But here’s the key thing you need to understand: LLM responses/answers are usually unique strings of text. As in: if you were to Google the full bit, in quotes, you wouldn’t find that paragraph or bullet list or whatever word-for-word somewhere on the internet. It’s usually not using a single source and repeating an answer exactly from a particular place. Each response is the culmination of all its combined learnings from all its training data (which is important to remember when we discuss copyright law).
Hence, the name “Generative A.I.”. It’s generating a response on-the-fly.
2. Why Does Google Care About LLMs?
Google makes money because the internet goes to search engines to get answers (whether that answer is another website, a product, a fact, idea, etc.) and because they’re the most popular search engine (92% market share).
They make 56% of their revenue (as of Q4 2022) from search ads.
So, if people were to stop going to search engines for answers, and/or stop using Google in particular, then their trillion dollar market cap will start to deteriorate. They have more to lose than anyone when it comes to changes in behavior for how, and where, users go to get answers on the internet.
Which is why, just 3 months after launching in November 2022, when Reuters reported ChatGPT had crossed over 100 million users (making it the fastest product to achieve that level of adoption in all of human history), Google must have been sweating. Profusely.
For the first time since becoming the most popular search engine in the world, Google seemingly has real competition. And ironically, when I Googled to find out when they first became the most popular search engine, they incorrectly say 2000. I then asked ChatGPT, which correctly told me 2004.
That example, along with so many others, leads us to the next big reason why Google must be sweating – it doesn’t take a rocket scientist to see just how good LLMs like ChatGPT already are for a lot of things.
NOTE: if you haven’t tried ChatGPT yourself – please – stop reading this, sign up and start asking it things. Ask it for advice with an issue you’re dealing with, or recipe ideas based on the ingredients in your fridge, or to write you a poem that’s tailored to your partner’s specific interests (more ideas here). Reading about it doesn’t do it justice – the longer you wait to try it out, the further you’ll fall behind.
Now don’t get me wrong – ChatGPT and other LLMs are far from perfect. In particular, they’re heavily criticized for “hallucinating”, or confidently saying incorrect things.
But between that Reuters report, what everyone can see with the naked eye, and with what Google’s own A.I. engineers must have been telling their own executives behind closed doors, it’s pretty obvious this emerging technology is a real threat to Google’s trillion dollar monopoly.
Which brings us to the innovator’s dilemma. Google doesn’t want to disrupt themselves. They hate what’s happening with generative A.I. and LLMs in particular. At the very least, it creates a lot of uncertainty around future revenue and profits. At the very most, it could destroy profit margins or kill their monopolistic hold on question and answering entirely.
But if they don’t disrupt themselves, the thinking is, someone else will. And ideally, this “disruption” happens as slowly as possible so they have time to adapt their search ads business with minimal negative impacts. But the slower they adapt, the more they risk becoming the next Blockbuster.
So, because of this existential threat and the dilemma they’re in, Google had no choice but to respond in some big, scary ways.
3. What Exactly Did Google Announce on May 10th?
May 10th, 2023 is the day Google held their I/O conference. With investors nervous about ChatGPT breathing down their necks, it’s understandable the conference focused heavily on A.I.
A lot of things were announced that day, but the announcement I previously highlighted was, in my opinion, the biggest. It included this (excruciatingly upbeat-sounding) 92 second video.
These “SGE” features shown are only in beta. They’re currently not shown to users who haven’t specifically signed up to start seeing them (by going here). But if and when that changes, there will be massive negative ramifications for global SEO traffic.
This is because, if you look closely at the example screenshots shown, you’ll see that information and answers are provided without citing any sources. And, as opposed to current Featured Snippets, they’re showing much larger amounts of content, much richer information and even clickable links for additional follow up searches (i.e. for items listed) so that you never have to leave Google (unless you’re clicking an ad).
Which means that, if you thought the current trend in zero-click searches was already bad, buckle up. It could get so, so much worse.
And as if that’s not crazy enough – Lily Ray pointed out on Twitter that this content is sometimes a word-for-word copy from a particular website and therefore literally not Generative A.I. That’s instead just a straight up un-attributed Featured Snippet and, arguably, a blatant violation of U.S. copyright law. Even if Google considers this particular issue a bug and fixes this from happening again (although the Google rep that responded by no means responded like it was), it shows us how irresponsibly fast the Web’s biggest gatekeeper is moving here.
Make no mistake: this is how a scared, trillion dollar incumbent acts when going through an existential crisis. And if you don’t believe they are, then take it from one of Google’s own researchers.
It’s impossible to know how quickly Google’s SGE feature will improve and be adopted (whether users opt-in or not). There’s also a glimmer of hope that Google might change course and start citing their sources in this new experience.
But with that said, given the environment they’re operating in, I wouldn’t count on anything at this point.
The Present: Important Additional Context You Should Know
That brings us to the present. But before we can start speculating about the future, we also need to understand:
- The Content Types and Keyword Groups That Will Be Disrupted the Quickest
- Where ChatGPT & Other LLMs Get Their Training Data From
- The Current State of A.I. Training & Copyright Law
- What the Competitive Landscape for the LLM Industry Currently Is
Where Do LLMs Currently Excel (and Not Excel)?
The distribution of disruption to online content providers by generative A.I. will be unique. It won’t all hit us the same. It will hit certain industries more than others. To understand why, it’s important to understand where LLMs currently do well, and where they fall short.
As of right now, today, LLMs are a way better experience than traditional search for:
- Idea generation – i.e. “recipes”, “places to go” and “fan fiction” are all examples of keyword groupings that focus less on objective truths and instead on possibilities that fit within certain guidelines.
- Information with low (to no) rate of change – i.e. “how to fill a nail hole in drywall”, “what happened at the battle of Gettysburg”, and “what is entrepreneurship” are all keyword examples that don’t really need much updating based on new information.
- Non-fringe topics / information that has been replicated in numerous places – i.e. there have been 1000s of articles previously written on the topic of “how to get over a breakup”. This gives LLMs lots and lots of practice to figure out a perfect answer during the training process. This is why I expect LLMs will impact B2C search way more than B2B.
On the flip side, the most popularly criticized LLM use case today is when they’re asked to provide objective truths in “high stake” situations.
An example of high stakes would be a law firm using it to find case law examples to cite in a lawsuit, or a doctor using it to diagnose cancer. In both situations, their users have a much greater need for certainty. Even if an LLM was right 95% of the time, they’d still want to fact check it (given the stakes), and therefore they wouldn’t really replace a traditional Google search with an LLM prompt, they’d end up just doing both. Which wouldn’t make LLMs that useful.
So in informational areas where the stakes are higher for users, I expect LLM disruption will take an extra year or two or five to reach them.
Where do ChatGPT & Other LLMs Get Their Answers From?
Every LLM, including ChatGPT, is only as good as its training data.
An LLM’s training data is the information it’s fed to “learn”. This could be things like scanned pages of books, statistical databases (i.e. stock prices) or scraped webpages (i.e. from Wikipedia).
While clean, non-public datasets can be a huge differentiator for LLMs, the last category (scraped webpages) is the most scalable and, as a result, what the majority of LLMs depend most on for generating their answers.
The only problem is, scraping the whole internet is hard. While Google and OpenAI have the necessary resources to do so, most open source LLMs do not. Instead, they most commonly turn to one of two publicly available datasets.
The first dataset comes from Common Crawl, a nonprofit that’s been scraping the Web since 2013. Over those 10 years, they’ve gotten really good at it – their March/April 2023 dataset contains 3.1 billion webpages from 34 million unique domains. Their mission to “democratize access to web information” was originally popularized for lowering the barrier to entry for new startup search engines to compete with Google. However, most mentions of them and their datasets these days are in relation to their use by LLMs for training purposes.
The second dataset comes from Google, the most experienced and successful web scraping company of all time. What’s interesting though is that the dataset they provide, known as the C4 dataset, is simply just Common Crawl’s data but cleaned up (meaning: a smaller index of webpages with low quality ones removed).
Google’s move is understandable – they won’t, and probably never will, just freely give away what is ultimately their trillion dollar secret: the database of webpages they’ve scraped and the answers they derive from that content. Instead, they can just clean up an existing dataset, barely giving away any of their special sauce, and get all of the social credit of contributing to open source.
In the end though, it’s worth noting that Google has one of the biggest long-term advantages for building an industry leading LLM. Their decades of experience scraping the web and their internal database of already-scraped-webpages makes them an early favorite to win out in the LLM wars to come.
Do LLMs Cite Their Sources?
No. ChatGPT, Google Bard and most other LLMs do not cite their sources.
Only Google Bard freely provides sources for an answer, but only after directly asking it for sources. For ChatGPT specifically, it takes clever prompt engineering to get it to provide any sources in any situation, and even then, it apparently hallucinates and gives made up sources often.
This isn’t necessarily as much ill intent as you might think. A core issue with LLMs is that they don’t even know exactly how they came up with their answer and, hence, where that answer came from. As of today, only Google and Bing have made intentional effort to curtail this characteristic (by sometimes opting to answer based on a specific high ranking source’s information instead of a normal, “pure” LLM response).
Here’s ChatGPT’s own explanation for why it doesn’t provide sources:
> “OpenAI’s ChatGPT is a machine learning model that was trained on a massive amount of text data from the internet. The specific sources of this text data are not retained in the model because the primary focus during the training process was to create a model that can generate coherent and informative text, rather than tracking the sources of the data used to train it. Additionally, keeping track of the sources for all the data used in the training process would require a significant amount of computational and storage resources”
Is My Website Being Used to Train LLMs?
One way to find out is by searching here to see if your website is included in Google’s C4 dataset. If it is, then it means your website’s content is being used to train numerous LLMs.
Note: if you’re wondering, the answer is “no” – there isn’t anything you can do about it. There is currently no way to formally request Google, or Common Crawl, to remove previously made copies of your website’s content from their indexes. The only thing you can do is block Common Crawl from making new copies.
A second way to find out is by asking it questions about your company, product, employees, etc that are only answered on your website and not elsewhere online.
What is the Current State of U.S. Regulation on Copyright & A.I. Training Data?
Note: I’m not a lawyer and this section does not constitute legal advice.
Congress issued a report on May 11th clarifying that we’re not sure yet if the current use of copyrighted works to train A.I. models is illegal:
> “A.I. companies may argue that their training processes constitute fair use and are therefore noninfringing…”
> “These arguments may soon be tested in court, as plaintiffs have recently filed multiple lawsuits alleging copyright infringement via A.I. training processes. On January 13, 2023, several artists filed a putative class action lawsuit alleging their copyrights were infringed in the training of A.I. image programs, including Midjourney and Stable Diffusion. The class action lawsuit claims that defendants “downloaded or otherwise acquired copies of billions of copyrighted images without permission” to use as “training images,” making and storing copies of those images without the artists’ consent. Similarly, on February 3, 2023, Getty Images filed a lawsuit alleging that “Stability A.I. has copied at least 12 million copyrighted images from Getty Images’ websites . . . in order to train its Stable Diffusion model.” Both lawsuits appear to dispute any characterization of fair use…”
While those lawsuits focus on copyrighted images, they should lead to some kind of legal precedent that applies to copyrighted text as well. The only issue is, lawsuits can take a while. The average class action lawsuit in the U.S. takes two to three years to resolve. So in the meantime, A.I. models will continue to train using copyrighted content, without anyone’s permission, whether we like it or not.
What is the Current State of the LLM Industry Landscape?
While ChatGPT (and ChatGPT alone) blazed the trail for widespread user adoption of LLMs, competition is quickly heating up.
Today there are now dozens of open-source LLM models. Some already perform better than ChatGPT, depending on the method of evaluation. One recently released open source model (MozaicML) performed quite competitively despite costing only $200,000 to train. Another (MLC) aimed to be so lightweight that it can be deployed on an iPhone. Those two examples, and many others, show that the core technology behind LLMs is quickly becoming commoditized.
On the opposite side of things (closed source), the most important one to watch is Google’s own LLM interface, Google Bard, which received a massive upgrade on May 10th. Bard can now check the Web for more recent info when its training data falls short (example). While ChatGPT’s Browsing plugin was created to do the same, Bard’s experience is much more seamless. You don’t need to tell it when it should do this – it figures out when it needs to do so all on its own. This feature, in my opinion, makes Bard arguably the most useful LLM currently available (for question and answering).
Important Note: U.S tech giants aren’t the only ones working on this. China’s Alibaba and Baidu are rapidly developing their own LLM models, too.
The Future: Wild Speculations About What Happens Next
Finally, the fun (or not so fun) part: making wild speculations about the future of our industry.
Here are the areas I’m thinking about in order to determine what possible best and worst case scenarios could play out (and their probabilities of happening).
- How Regulation on Copyright & A.I. Training Data Will Play Out
- Whether or Not LLMs Continue to Rapidly Improve Even Further
- Whether New Users Will Start Adopting LLMs en Masse
- What Moves Google Might Make Next
- What We as Individuals and as a Collective Do in Response to the Threat of A.I.
Will Copyright Holders Get Regulatory Protection?
Whether our government chooses to regulate A.I. or have a “laissez-faire” approach will have a huge impact on the futures of all content creation industries (i.e. music, photography, art, etc.), not just our own.
On one hand, massive amounts of job loss could result in the necessary political pressure to force regulators to step in. While this scenario is more reactive than proactive, I think it’s the best argument in the “hopeful for regulation” camp.
On the other hand, I’m personally not too hopeful for these main reasons:
- What didn’t happen with crypto – the crypto industry has been asking for clear regulation for years. Despite the industry’s decade plus of existence and current trillion dollar plus market cap, regulators continue their reluctance in providing any kind of clear regulatory guidance.
- What was (and wasn’t) said in the OpenAI congressional hearing – if you check the transcript, the word “copyright” was barely mentioned (only 8 times out of ~30,000 spoken words). Instead, the hearing mainly focused on A.I. licensing and safety measures and how A.I. related to each of the representatives personal agendas.
- Competition from global political powers – stopping or slowing down A.I. development in the U.S. will just mean that tech giants in other countries get ahead. Japan just announced they won’t protect copyright holders from A.I. training. Expect China, who always has shown little to no regard for copyright law, to do the same. On the bright side, the European Union seems to be on the precipice of new protections. But overall, while it’s impossible to know how this unfold, there is no way that regulators aren’t weighing the factor of famling head the advacements made by major politocal leaders.
If They Do, When Might That Happen?
Even if regulatory protection happens, it could be too little, too late for those who currently have the most to lose.
For example, if you’re a stock photographer, you might be struggling for work right now. You don’t have 2 years to wait for regulation to protect your industry. I can’t help but think that Shutterstock, Getty Images and other companies will incur massive layoffs well before their pending lawsuits get resolved.
If They Do, What Might the New Rules Be?
The most obvious rule (in my personal opinion) that regulators could put in place would be getting the consent of copyright holders to use their works to train A.I. models.
But at this stage it’s really impossible to say what the rule(s) would be.
Maybe A.I. companies will only need to inform copyright holders that their work is being used? Or maybe just allow for copyright holders to manually request their works not to be used (like a DMCA takedown request)? Or maybe different types of content are handled differently (like text content being OK to use if properly cited as a source alongside the answer)?
Will LLMs Continue to (Rapidly) Improve?
If you’ve been paying close attention to generative A.I. and LLMs in particular since the second half of 2022, you might have had the same reaction of absolute disbelief that I had when ChatGPT 4 was released.
I reacted that way because ChatGPT 3.5, which on its own was a huge improvement on the previous model, had come out less than 5 months earlier.
It doesn’t look like that rate of pace will continue (at least in the short-term) – in April, Sam Altman said they hadn’t yet started training a model 5, making a release not too likely to drop prior to the end of 2023.
It’s impossible to know where we’re at on the curve of technological progress with generative A.I. and LLMs specifically.
Past technological hype cycles have shown us that short periods of rapid progress can be followed by years of little to no progress, such as with self-driving cars and virtual reality. Both examples made a lot of progress between 2010-2016, giving rise to grand predictions of mass adoption that would soon follow but didn’t exactly pan out.
How Might LLMs Continue to Improve?
It’s important to consider the ways that LLMs might continue to improve and for which particular use cases they will improve most for next.
At the very least, LLMs could meaningfully improve without any changes in the underlying technology. This is because, if you remember, LLMs are “only as good as their training data”. That statement is bi-directional. We could see factual accuracy increase from ChatGPT and others simply from the cleaning of existing datasets or through training on entirely new datasets.
(And if popular LLMs were to ever start training on customer data, we could see another factor of magnitude improvement in quality.)
How Else Might the Training Data Landscape Change?
Reddit and Stack Overflow have both declared they’re cool with letting A.I. models train on their user’s content, as long as A.I. companies pay for that right to do so.
I expect every major UGC site will follow their lead and have the same stance. Think: Twitter, Yelp, etc.
Which creates a very unfortunate dynamic for professional content creators: if the information contained in their content has been created and left by users on platform websites (i.e. Amazon reviews, Reddit comments, Facebook posts, etc.), that content will probably be unapologetically used against them.
Which means that, even if copyright holders win the legal right of consent for training A.I., UGC platforms will happily line up to cut deals with A.I. companies and sell everyone out.
For example, if Amazon decided to license their customer review data to Google for training their LLM, all Amazon affiliate sites would essentially be screwed. Because it means Google would then be able to provide no-attribution-needed content directly on SERPs (i.e. like this) in a way that’s not legally or ethically questionable long-term.
How Will the User Adoption of LLMs Play Out From Here?
Even if the tech and user experience of LLMs gets unanimous acclaim as being objectively better than traditional web search, it doesn’t guarantee new users will stop using Google.
According to the founder of Neeva, a now-defunct startup search engine, the hardest part of mainstream adoption of LLMs might not have anything to do with the tech or UX:
> “Throughout this journey, we’ve discovered that it is one thing to build a search engine, and an entirely different thing to convince regular users of the need to switch to a better choice,”
> “Contrary to popular belief, convincing users to pay for a better experience was actually a less difficult problem compared to getting them to try a new search engine in the first place.”
So not only does Google have a huge advantage in the coming LLM wars due to its extensive experience with web scraping, they also have what’s likely the biggest advantage of all: pre-existing mass adoption by an incredibly sticky user base. This is why, out of all scenarios, it’s most likely that users will “adopt” LLMs without a single change in behavior by continuing to go to Google.com but getting generative A.I. content served to them instead of featured snippets.
But, let’s put that scenario aside for a second and consider the adoption of LLMs beyond Google’s SGE feature.
At What Rate Might ChatGPT & Other LLMs Win User Adoption?
To assess how likely native LLM interfaces are to gain mainstream user adoption (and at what pace they might do so at), let’s consider arguably the best thing ChatGPT (currently) has going for it: Plugins.
Plugins allow developers to integrate ChatGPT with their products, essentially functioning as ChatGPT’s app store and increasing ChatGPT’s utility by an order of magnitude.
ChatGPT can now solve complex math equations, order groceries and talk to all your favorite work and non-work apps. And those capabilities are just a few examples of what’s to come. Most developers that signed up to start building their own Plugin are either still on the waitlist or only recently got off it.
This is why you can’t write off LLM interfaces as Google replacements. Because so far, we’ve barely seen what they’re capable of. With Plugins, ChatGPT could completely change the game of question and answering by allowing users to then do things with that information without any break in between.
Imagine this LLM prompt: “look up the best 10 sushi restaurants in New York, call them to see if they have any open reservations for Friday night, then text my girlfriend the list of available options, which times are available to book and pictures of their menus.”
In a future where that prompt is flawlessly executed, information gathering is just one piece of the application’s directive. The information gathering process essentially becomes invisible to the user. That future is what Google should most be scared of.
Will Users Trust LLM Answers?
Mainstream user adoption of LLMs requires users to trust their answers in two important ways:
- Factual accuracy
- Bias
The first will be much easier for ChatGPT and others to deal with long-term. This is because factual accuracy is (relatively more) measurable. There’s most likely some kind of break-even point where users are OK with the rate of error, at which point users could start being convinced that they can now blindly trust responses as being objectively true.
The other type of trust, bias, is much thornier to future user adoption of LLMs. If users are skeptical of answers and think LLMs are trying to manipulate them, it might be incredibly difficult to change their minds.
Enter: the U.S. mainstream media.
How the media covers ChatGPT and other generative A.I. tools in the near future will most certainly play a big role in their adoption. We’ve barely seen the potential of this so far but I believe this could be the biggest dark horse to mainstream adoption and could change everything on a dime.
Imagine negative anecdotal headlines like:
- “ChatGPT caught spreading misinformation about new statewide book ban.”
- “Racist logo for kids’ soccer team was ‘Midjourney’s idea, not mine, I didn’t even notice,’ says local mom.”
- “Dog owner trusted ChatGPT to find out if their beloved pet, Snickers, could safely eat avocados. ChatGPT was wrong and Snickers is now gone.”
While only 1 example (the first) attempts to stoke fears of intentional bias, all are headline types that could sway public sentiment on A.I. tools before they even try them.
What Are Google’s Next Moves?
Undoubtedly, the biggest question surrounding A.I.’s future impact on our industry is the potential rollout of Google’s new SGE feature (in its current form).
On that front, it’s important to consider the following:
- Will a meaningful number of users find out about and opt-in to SGE?
- Will Google reverse course on providing clearer sources/citations for answers?
- How quickly might Google roll out SGE for non opt-in users?
- Will Google roll out SGE content for some query types vs others?
The answer to those questions are anyone’s guess. I’ve barely formed any kind of real opinion of substance on any of them so, for now, I’ll keep my thoughts to myself.
Instead, I want to focus on another developing Google situation worthy of a place on your radar.
How Will Google Crawl the Web in the Future?
The best thing going for Google Bard at the moment is its ability to scrape the web for more recent information when it needs to (i.e. when it’s asked to summarize a recent event).
Since Google Bard is scraping a website and providing little to no benefit to that website in return (by stealing a potential web visit and not even crediting it as a source without asking), the owner of that website might wisely wonder: “Should I block Google Bard from crawling my website?”
For now, I believe the answer to that is a resounding “Yes”. Don’t let them eat your lunch for free.
But what if it wasn’t that simple?
Consider this terrifying reality:
Does Google crawl websites for Search and Bard using the same crawling agent name? Meaning, in that scenario, that it would be impossible to block Bard from crawling your website without also de-indexing yourself?
While I’m not 100% sure what the answer to that question is today, I did find coincidental evidence that indicates Google isn’t currently doing this.
On April 20th, just a few weeks before Bard rolled out live web scraping, Google launched “googleother”, a new crawling agent. It’s currently unclear if this agent name is what Bard is crawling under. There is nothing in that release that actually indicates when Google uses that agent. Their own support docs also have zero mentions of “Bard”.
Whether or not Google is currently crawling for Search and Bard under different crawling agent names is, to be frank, not too important. Bard has barely any user adoption at this point.
But what if that were to change? What if Bard goes neck-and-neck with ChatGPT in terms of users? If it does, Google has a trap card in its back pocket to extort the Web into playing ball with it while giving back increasingly less value to the websites it’s pulling answers from.
How Will We as an Industry Respond to the Threat of A.I.?
Lastly (and probably of least importance, unfortunately), it’s time to consider how we individually and collectively respond to this growing threat.
Will the threat of A.I. be taken seriously in our industry (by listening more closely to these people), or will we continue to be dismissive of technology that’s currently being compared to nuclear weapons?
If we do finally take it seriously, what will we do in response?
- As individuals, will we choose to block Common Crawl, Googleother (Bard), ChatGPT’s Browsing plugin and others in our robots.txt files?
- As a group, will we support organizations like the Human Artistry Campaign that are fighting for our rights as copyright holders?
I have no idea what the answers to those questions are. I do hope, though, that more of us are now at least considering them.
Special thanks to Kai Isaac, Paul May, Ryan Mclaughlin, Grant Merriel and Alan Morte for the discussions that lead to the takeaways in this article.